Latent Class Modeling
Latent class modeling refers to a group of techniques for identifying unobservable, or latent, subgroups within a population. Researchers have developed and expanded methods like latent class analysis (LCA) and latent transition analysis (LTA) over the last two decades. Our current research focuses on expanding methods to include latent class variables in larger models of complex developmental processes. Latent class analysis (LCA) identifies unobservable subgroups within a population. We work to expand LCA models to allow scientists to better understand the impact of exposure to patterns of multiple risks, as well as the antecedents and consequences of complex behaviors, so that interventions can be tailored to target the subgroups that will benefit most. Latent transition analysis (LTA) is a related method that allows scientists to estimate movement between subgroups over time.
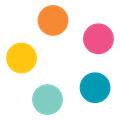
Let’s stay in touch.
We are in this together. Receive an email whenever a new model or resource is added to the Knowledge Base.