Static
All of the models presented here are considered “static” in the sense that they model a single categorical latent variable where an individual’s class membership does not change. These models include latent class analysis (LCA), latent profile analysis (LPA), and mixed indicator models. Although different names for these models appear throughout the literature, here we use the convention that models that include only categorical indicators are LCAs, include only continuous indicators are LPAs, and include both categorical and continuous indicators are mixed indicator latent class models.
Dynamic
All of the models presented here are considered “dynamic” in the sense that they model change over time in a categorical latent variable; that is, an individual is allowed to transition between latent classes when longitudinal data are collected. These models include latent transition analysis (LTA). A “traditional” perspective on LTA is used here, so that these models include LTAs with the same classes and indicators at all times. More general models are available in our collection of “advanced” models. Note that different names for these models appear throughout the literature, a common one being latent Markov models. In addition, more recent literature refers to the identified classes as “classes” whereas older literature often refers to the identified classes as “statuses” to convey the dynamic nature of membership.
Advanced
All of the models presented here add advanced features to “baseline” static and dynamic latent class models. This includes information about latent class moderation, adding inverse propensity score weights to models, multilevel latent class models, and associative latent transition analysis, among other topics. Note that these models fall under the broad classification of loglinear modeling with latent variables.
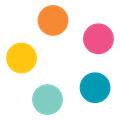
Let’s stay in touch.
We are in this together. Receive an email whenever a new model or resource is added to the Knowledge Base website.