Description
Latent class analysis (LCA) and latent transition analysis (LTA) can be extended in a variety of ways. Advanced features, such as covariates, outcomes, moderators, and inverse propensity score weights, can be added to “baseline” static and dynamic latent class models. All of the models discussed here add advanced features to baseline models. Over time, these pages will includes information about recent research on latent class moderation, adding inverse propensity score weights to models, multilevel latent class models, and associative latent transition analysis, among other topics. Note that these models all fall under the broad classification of loglinear modeling with latent variables.
Extensions to Baseline LCA and LTA
LCA with a distal outcome
Latent class membership can be used to predict an outcome. There are a number of competing approaches proposed in the literature, but the “BCH approach” is currently recommended in most applications. This approach can be extended to include control variables in the regression model predicting the outcome.
Latent class moderation
Moderation analysis is typically conducted by incorporating a single variable (e.g., gender, baseline severity) as a moderator into a multiple regression model. By using LCA, researchers can identify subgroups of people exposed to a common set of factors and who, therefore, may respond differently to intervention. That is, latent class membership can be used as the moderating variable in multiple regression.
LCA with causal inference
LCA with covariates models the association between classes and predictors, but causation cannot be inferred unless people are randomly assigned to levels of the predictors of latent class membership. Modern causal inference methods, such as inverse propensity weighting, can be used to adjust for potential confounding in observational data.
Advanced Models
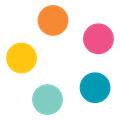
Let’s stay in touch.
We are in this together. Receive an email whenever a new model or resource is added to the Knowledge Base.