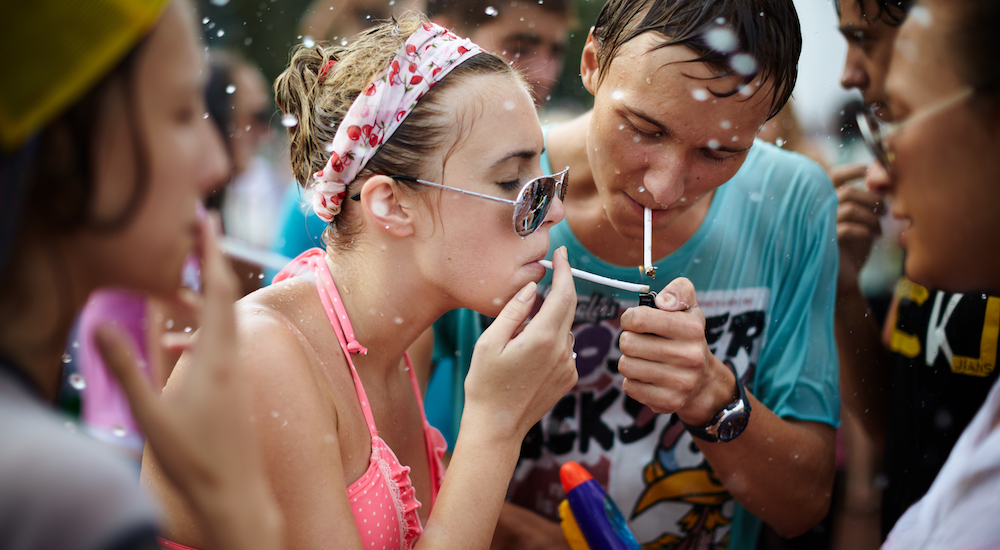
This example is based on publicly available data from the Youth Risk Behavior Surveillance System. If you have experience analyzing data, you can download the data and PROC LCA and perform the analysis yourself. This example is explained in detail in chapter 2 of Latent Class and Latent Transition Analysis by Collins & Lanza (2010). This analysis allowed the research team to identify complex behavior patterns and variables that predict high-risk behavior patterns, as well as identify the subgroups of youth who are most at-risk for negative health consequences.
About these Data
- Measured 12 heath-risk behaviors
- 13,480 US high school students (grades 9 – 12)
- Collected in 2005
Participants responded to questions about sexual behavior, smoking behavior, alcohol-consumption behavior, and previous usage of other prevalent drugs, including marijuana, ecstasy, and cocaine. The 12 questions in the table below were the “items” used to identify the latent classes.
So, by looking at the table to the right, you can see that 25% of the students had five or more drinks in a row during the month prior to data collection. Also, 7% had sex before the age of 13. This information is interesting and potentially useful on its own, but it might be more useful if we could see common patterns of behavior among groups of students.
LCA Mathematical Model
The analysis was completed using a SAS procedure developed by The Methodology Center, PROC LCA. PROC LCA is easy to use and requires minimal syntax.
Selecting the Proper Number of Classes
To select the number of classes for the model, specify and run a 2-class model and repeat with 3 classes, 4 classes…, up to the highest plausible number of classes. From the results, information about fit (including log likelihood, degrees of freedom, G2, AIC, BIC, CAIC, etc.) are compared to identify the optimal model. Also, the bootstrap likelihood ratio test can be used to compare models. The Methodology Center created a SAS macro to perform the bootstrap likelihood ratio test for PROC LCA users.
Results
In LCA, the responses of all participants to all items are analyzed. A specified latent class model is fit to the data, and the parameter estimates are obtained. Once the number of classes is selected, the output includes the probability of a response to EACH risk item in the inventory for each latent class. In other words, you will see the probability that members of each class had of engaging in each risky behavior. (See the table below.) For this analysis, a five-class model was selected, which means that the analysis revealed five latent subgroups in the population of teens. The scientists interpreted the results and assigned the following labels to the groups:
- 67% of the respondents fell into the Low Risk class.
- 14% were in the Binge Drinkers class.
- 9% were in the Early Experimenters class.
- 5% were in the High Risk class.
- 4% were in the Sexual-Risk Takers class.
Note that the totals add up to 100% (within rounding) because in theory, every individual belongs to one and only one class.
But what do these categories mean, and how were the labels arrived at? Below is the table of item-response probabilities, which indicate the likelihood that teens in a given class reported in engaging in a particular risky behavior. These probabilities provide the basis for labeling the classes.
The analysis reveals the classes; the researcher interprets and labels them.
All responses where a group member was more likely to reply “yes” are indicated by a number larger than .50 (.50 would indicate half of the group members said “yes” and half said “no”). So .04 next to “Smoked first cigarette before age 13” in the Low Risk column means that members of the Low Risk group had a 4% chance of saying they had smoked prior to age 13. The table shows that members of the Low Risk group were very unlikely to report any risk behavior, but their most prevalent behavior is having had an alcoholic drink before age 13 (14%). Members of the Binge Drinkers group were most likely to have had 5 or more drinks on one occasion (74%) but were also significantly more likely to have driven while drinking (42%) than the Low Risk group (1%). Early Experimenters had a high probability of experimenting with alcohol and tobacco before age 13, but they had a lower than 50% chance of participation in all other risks. Still, Early Experimenters had a higher likelihood of engaging in each risk behavior than members of the Low Risk group. This analysis provides information about the combinations of risks youth are likely to be exposed to, and the proportion of youth exposed to the risks.
The analysis provides information about patterns of risky behavior and the prevalence of those patterns.
NOTE: The names of the groups were assigned by the scientists based on the results of the LCA. The analysis divides the groups empirically; scientists label the groups based on what the groups indicate about the data.
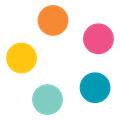
Let’s stay in touch.
We are in this together. Receive an email whenever a new model or resource is added to the Knowledge Base.