Description
Latent class modeling refers to a group of techniques for identifying unobservable, or latent, subgroups within a population. Researchers have developed and expanded methods like latent class analysis (LCA) and latent transition analysis (LTA) over the last two decades. Our current research focuses on expanding methods to include latent class variables in larger models of complex developmental processes. Latent class analysis (LCA) identifies unobservable subgroups within a population. We work to expand LCA models to allow scientists to better understand the impact of exposure to patterns of multiple risks, as well as the antecedents and consequences of complex behaviors, so that interventions can be tailored to target the subgroups that will benefit most. Latent transition analysis (LTA) is a related method that allows scientists to estimate movement between subgroups over time.
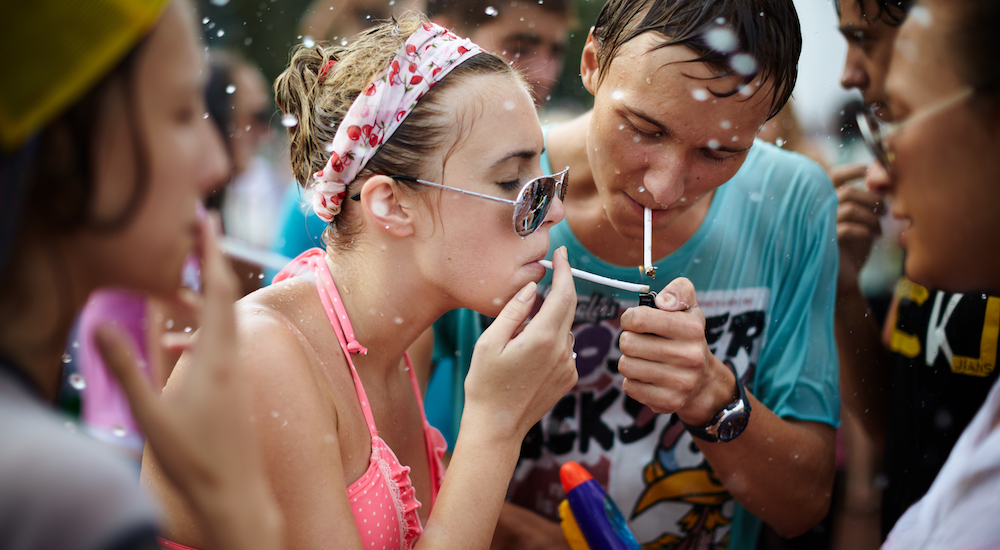
LCA Introductory Example: Profiles of Teen Sex and Drug Use
In this example, LCA identifies five subgroups of teenagers based on their substance use and sexual behaviors. The latent variable “youth risk behavior” is measured by the observed variables “sex,” “drinking,” “smoking,” and “other drugs.” This analysis allows us to identify complex behavior patterns and variables that predict high-risk behavior patterns, as well as identify subgroups of youth who are at-risk for negative health consequences. With this information, scientists can develop interventions that target individuals with the greatest need.
Read more here.
Resources
There are a variety of resources available to help you learn more about LCA. See our Resources page for the following:
Static Models
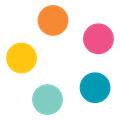
Let’s stay in touch.
We are in this together. Receive an email whenever a new model or resource is added to the Knowledge Base.