The models presented here include indicators all or some of which have categorical response options.
LCA: Adding outcomes using an adjusted 3-step approach (automated, BCH)
Description This code adds a binary and a continuous outcome to the 4-class baseline LCA model with all binary indicators from Exercise 1 in the linked page. The binary outcome is political beliefs (not conservative vs. conservative) and the continuous outcome is the number of evenings out per week (average 0-7). This code uses the automated 3-step BCH approach as we explore the association between latent class membership and each outcome. Software Downloads Mplus Exercises Exercise 1 This exercise asks you to fit a 4-class, latent-class model for marijuana use and attitudes using 7 binary indicators of the latent class...
LCA: Adding outcomes using an adjusted 3-step approach (manual, BCH)
Description This code adds a binary and a continuous outcome to the 4-class baseline LCA model with all binary indicators from Exercise 1 in the linked page. The binary outcome is political beliefs (not conservative vs. conservative) and the continuous outcome is the number of evenings out per week (average 0-7). This code uses the the manual 3-step BCH approach as we explore the association between latent class membership and each outcome. Software Downloads Mplus Exercises Exercise 1 This exercise asks you to fit a 4-class, latent-class model for marijuana use and attitudes using 7 binary indicators of the latent...
LCA: Baseline LCA with 3+ level categorical indicators
This code fits a longitudinal latent class model, using categorical indicators with 3+ levels, to identify latent classes indicated by multidimensional experiences of racism and heterosexism during the transition to adulthood among sexual minority men of color.
LCA: Baseline LCA with all binary indicators
This code fits a 4-class, baseline, latent-class model for marijuana use and attitudes using 7 binary indicators of the latent class variable. This code also plots the item-response probabilities using a line graph.
LCA: Latent class moderation
This code demonstrates how to use a latent class moderator to examine heterogeneity in intervention effects among adolescents receiving treatment for cannabis use. First, the code identifies latent classes of contextual and individual risk at baseline using LCA. Then, it uses an adjusted 3-step approach with BCH weights to regress the outcomes on level of care, latent class membership, the interaction between them, and covariates.
LCA: LCA with a covariate (1-step approach)
This code fits a 4-class, latent-class model for marijuana use and attitudes using a model-based approach (1-step approach). It includes a covariate for grades in the model.
LCA: LCA with a covariate and a grouping variable (1-step approach)
This code fits a 4-class, latent-class model for marijuana use and attitudes using a model-based approach (1-step approach). It includes a covariate for grades and a grouping variable for year in the model.
LCA: LCA with a grouping variable and measurement invariance
This code fits a 4-class, latent-class model for marijuana use and attitudes using 7 binary indicators of the latent class variable. It includes a grouping variable for year, and observations came from 3 different years.
LCA: LCA with a grouping variable and without measurement variance
This code fits a 4-class, latent-class model for marijuana use and attitudes using 7 binary indicators of the latent class variable. It includes a grouping variable for year, and observations came from 3 different years. Measurement invariance across groups is not imposed resulting in an unrestricted latent class model with multiple groups.
LTA: Baseline Latent Transition Analysis with Categorical Indicators
This code fits a 4-class, latent-class model for marijuana use and attitudes using 7 binary indicators of the latent class variable. It includes a grouping variable for year, and observations came from 3 different years. Measurement invariance across groups is imposed such that analogous item-response probabilities within classes are restricted to be equal to each other across groups.
LTA: Baseline LTA with 2 times, all binary indicators, and measurement invariance
This code fits a 2-time, 5-class, latent-transition model for delinquency over time using 6 binary indicators of the latent class variable. Measurement invariance across time is imposed such that analogous item-response probabilities within classes are restricted to be equal to each other across times.
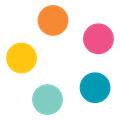
Let’s stay in touch.
We are in this together. Receive an email whenever a new model or resource is added to the Knowledge Base website.